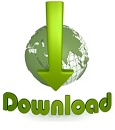
From the earlier investigations I had identified the three categories of knowledge grounded in KBpedia that could act as bases or features to machine learning namely, structure, annotations and pages.
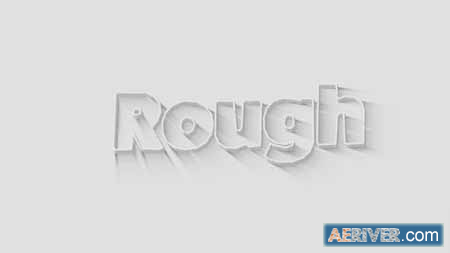
PyTorch ArchitectureĪ critical question in contemplating this plan was how exactly data needed to be produced, staged, and then fed into the analysis portions. For example, knowing I wanted to compare results across algorithms meant I needed to have a good understanding of testing and analysis requirements before starting any of the tests. Some of these steps also needed some preliminary research before proceeding. Discuss basic test parameters/’gold standars’.Introduce the trics module and confusion matrix, etc.Text summarization for short articles (gensim).Clean Wikipedia articles, all KB annotations.Obtain Wikipedia articles for matching RCs.The listing below summarizes these steps, and keys the transition point (as indicated by CWPK installment number) for proceeding to each next new installment: But, in total, I formulated about 30 tasks going forward that appeared necessary to cover the defined scope. I will weave the results of this research as the next installments unfold, providing background discussion in context and as appropriate. But I needed to understand the capabilities now available to us with Python, so I also studied each of the candidate keystone packages in some detail. I revisited each of these use cases and got some ideas of what made sense for us to attempt with Python.
#Clean text with gensim series#
It is true, for example, that we had already prepared a pretty robust series of analytic and machine learning case studies in Clojure, available from the KBpedia Web site. Much reading and research went into this effort. And, if so, how shall the efforts be sequenced and what is the flow of data? So, with an understanding of how we could extract general information from KBpedia useful to analysis and machine learning, I needed to project out over the entire anticipated scope to see if, indeed, these initial sources looked to be the right ones for our purposes. But, how all of this was to unfold, what my plan of attack should be, became driving factors I had to solve to shorten my development and coding efforts. I had done an adequate initial diligence. The broad ecosystem of Python packages I was considering looked, generally, to be good choices to work together, as first outlined in CWPK #61. These three sources of structure, annotations and pages are the input basis to creating our own embeddings to be used in many of the machine learning tests. Our coding efforts in this installment will obtain and clean the Wikipedia pages that supplement the two structural and annotation sources based on KBpedia that were covered in the prior installment. We discuss general sources of data and corpora useful for machine learning purposes.
#Clean text with gensim install#
We describe the additional Python packages we need for this work, and install and configure the first ones.

We provide particular attention to the architecture and data flows within the PyTorch framework. In this particular installment we flesh out the plan for completing these installments and discuss data sources and completing data prep needed for the plan. We conclude this Part VI with a summary and comparison of results across these installments based on the task of node classification. We next devote four installments to deep learning, split equally between the Deep Learning Graph ( DGL) and PyTorch Geometric ( PyG) frameworks. Then we devote two installments to ‘standard’ machine learning (largely) using the scikit-learn packages. We devote two installments to data sources and input preparations, largely based on NLP (natural language processing) applications. We will be devoting our next nine installments to this area.

With our discussions of network analysis and knowledge extractions from our knowledge graph now behind us, we are ready to tackle the questions of analytic applications and machine learning in earnest for our Cooking with Python and KBpedia series.
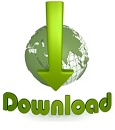